Determining the Optimal Benchmark for Funds
Ask the asset manager?
SUMMARY
- Identifying the right benchmark for a fund or portfolio can be difficult
- Many common metrics like correlation or betas do poorly for benchmark selection
- Combining metrics is more effective
INTRODUCTION
Is gold the right benchmark for gold miners? Although these companies focus on excavating the precious metal, there are plenty of operational issues like staff strikes, collapsing mines, or politics that can affect these beyond the changes in the gold price.
How about REITs? They own real estate where valuations move at a glacial pace, but trade as public companies and are therefore subject to the whims of stock market investors, of which some only care about their daily P&L.
Capital allocators can ask the asset managers for the most appropriate benchmarks for their investment products, but fund managers have an incentive to select the ones that make them look best, so are biased. Choosing benchmarks manually is challenging as there are more than 3 million indices, so automating the benchmarking process is more sensible.
In this research article, we will evaluate a few simple approaches for systematically selecting the most appropriate benchmarks.
METHODOLOGY
We select 10 random funds that represent various asset classes like gold and strategies like momentum.
Theoretically, we could use the 3 million indices as the data set for benchmarks, but most of these are unknown and do not represent what most investors consider as benchmark indices. Given this, we select 40 well-known indices like the S&P 500 and aim to select one of these as the optimal benchmark for each of the 10 funds based on different metrics. We use a one-year lookback for all metrics, which is not particularly long, but does allow us to consider ETFs and mutual funds that were listed recently.
CORRELATION
The first approach we utilize is simply to calculate the one-year correlation of each of the 10 funds and then select the benchmark index with the highest correlation as the most appropriate.
We evaluate the results on a discretionary basis and argue that in four cases the benchmarks seem inappropriate. For example, the Russell 1000 Value Index is selected for MFS Total Return Fund (MTRCX), which does have a focus on cheap stocks, but also holds bonds. An index like the S&P Target Risk Growth Index that tracks a portfolio of stocks and bonds would have been more suitable but was not chosen.
It is worth noting that we only have one short-biased index in the data set, therefore the Inverse S&P 500 Index can be considered appropriate for the Short MSCI EAFE ETF (EFZ).
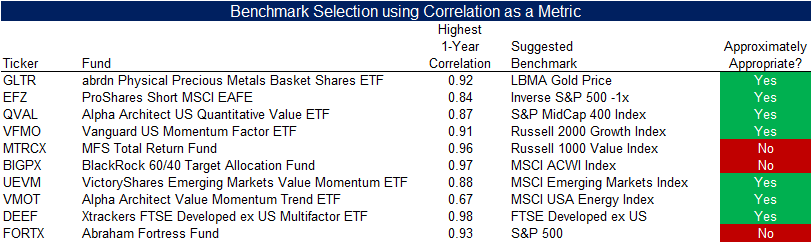
Source: Finominal
BETAS
Second, we compute the betas of the benchmark indices to the funds and select the ones whose beta is closest to one as the best benchmark. Betas provide the magnitude of the move of the fund compared to the benchmark index, while correlation measures the directional relationship.
However, this approach seems appropriate for only four funds. Some cases seem to be odd, but not necessarily wrong, eg the Abraham Fortress Fund (FORTX) holds stocks and bonds, so a high yield index was chosen as the benchmark, the MSCI China Index was selected for a precious metals fund (GLTR), and a commodities index was picked for a momentum fund (VMOT) that is currently overweight energy stocks.
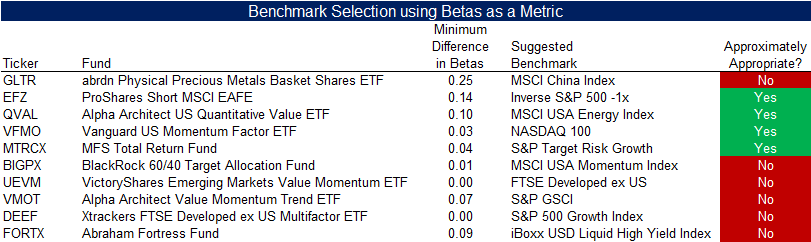
Source: Finominal
R2
Next, we calculated the R-squared of all indices for each fund via a simple regression analysis and select the index with the highest R2 as the best benchmark. Although slightly better than using betas, four benchmark selections seem questionable. The most prominent error is taking the MSCI EAFE Index as the benchmark for the ProShares Short MSCI EAFE ETF (EFZ), where the direction is ignored (read Factor Exposure Analysis 102: More or Less Independent Variables?).
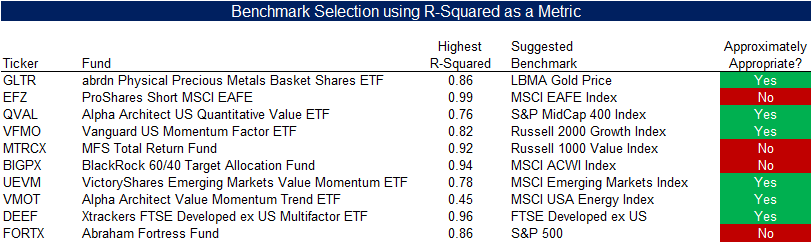
Source: Finominal
FACTOR EXPOSURE ANALYSIS
Following, we run a factor exposure analysis for each of the benchmark indices and 10 funds, where the index with the lowest absolute difference to the fund is selected as the most appropriate benchmark. The regression utilizes four asset classes and five equity factors as independent variables (read Mirror, Mirror, on the Wall, which is the Fairest Benchmark of All? and Sector vs Factor-based Benchmark Selection).
It is worth noting that this approach works well when trying to identify similar securities within a large data set. However, with only 40 benchmark indices this is less effective and three selections can be questioned, eg selecting a high yield index for the MFS Total Return Fund (MTRCX). Given that MTRCX’s portfolio is comprised of stocks and bonds this is not totally unreasonable, but does not seem perfect either.
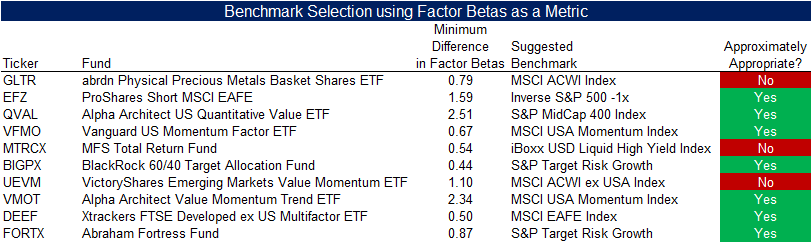
Source: Finominal
TRACKING ERROR
Finally, we evaluate using the tracking error to select an appropriate benchmark, which results in nine selections that seem reasonable. The odd one is a corporate bond index for a momentum fund (VMOT), which is simply a coincidence. However, the correlation between these two time series is zero, so we compute the ratio correlation to tracking error that results in benchmarks that are approximately appropriate for all ten funds.
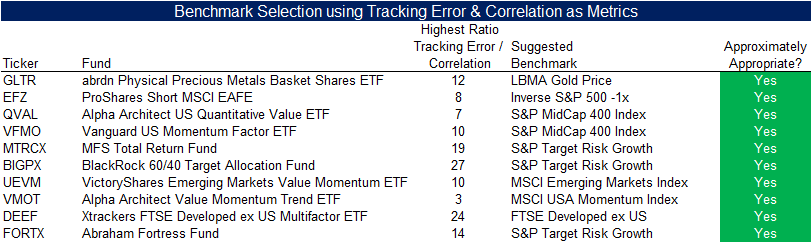
Source: Finominal
FURTHER THOUGHTS
As often in quantitative finance, magic happens when metrics are combined. Diversification benefits are not only generated when assets are combined, but also in stock selection, risk management, and benchmarking.
However, although we found one approach that selects reasonable benchmarks for the funds in this sample, there are certain types of products where a systematic approach will struggle, eg what is the right benchmark for a CTA or an equity market neutral fund? Perhaps these can only be benchmarked to themselves, or simply to cash interest rates.
RELATED RESEARCH
When Hindsight Becomes Foresight: Replicating Investment Performance
Replicating Popular Investment Strategies with Equities + Cash
Sector vs Factor-based Benchmark Selection
Mirror, Mirror, on the Wall, which is the Fairest Benchmark of All?
Factor Exposure Analysis 101: Linear vs Lasso Regression
Factor Exposure Analysis 105: Sectors versus Factors
Time Machines for Investors
Factor Exposure Analysis 102: More or Less Independent Variables?
ABOUT THE AUTHOR
Nicolas Rabener is the CEO & Founder of Finominal, which empowers professional investors with data, technology, and research insights to improve their investment outcomes. Previously he created Jackdaw Capital, an award-winning quantitative hedge fund. Before that Nicolas worked at GIC and Citigroup in London and New York. Nicolas holds a Master of Finance from HHL Leipzig Graduate School of Management, is a CAIA charter holder, and enjoys endurance sports (Ironman & 100km Ultramarathon).
Connect with me on LinkedIn or X.
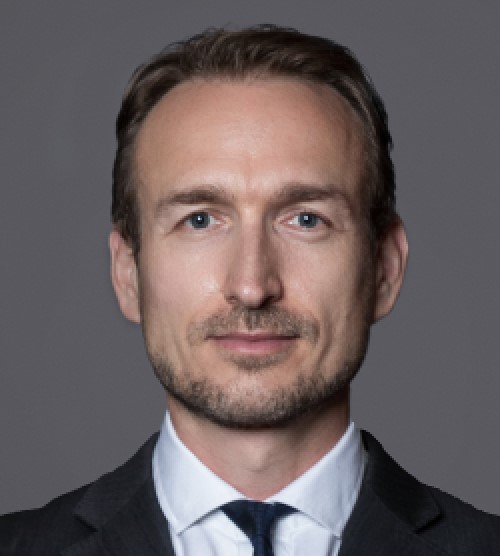