Hedge Fund Battle: Discretionary vs Systematic Investing
Man versus machine
November 2020. Reading Time: 10 Minutes. Author: Karl Rogers & Nicolas Rabener.
SUMMARY
- Given alternative data, machine learning, and AI advances, systematic should beat discretionary investing
- However, the performance of systematic and discretionary equity market neutral hedge funds has largely been the same since 2009
- Both were also correlated to the stock market, offered low returns, and featured no performance consistency
INTRODUCTION
Anyone who has watched the hit TV show ‘Billions’ will have noticed that the hedge fund billionaire Bobby Axelrod and his former lieutenant, and occasional nemesis, Taylor Mason have totally different trading styles. Bobby, although mathematically gifted, is a discretionary trader that makes investment decisions on the fly based on receiving new information on stocks from his analysts and network of colorful relationships. In contrast, Taylor is a traditional quant who believes in systematic trading.
For a quant, discretionary decisions are anathema and it is all about scientific research, data, coding, and following the model. While discretionary investing has not really changed significantly over the last few decades, the opportunity set for quants has improved dramatically. Computing power and data have grown exponentially. And then there is alternative data, machine learning, and artificial intelligence that have taken the headlines of industry webinars and conferences.
It is not difficult to view quants and systematic approaches to investing as vastly superior to old school discretionary investing. The latter can be seen as just another source of alpha to be exploited, similar to the retail crowd. In the battle between man versus machine, the latter should clearly dominate in this technology-driven age (read Quant Strategies: Theory vs Reality).
But do they?
In this short research note, we will compare the performance of discretionary versus systematic hedge funds.
DISCRETIONARY VERSUS SYSTEMATIC EQUITY MARKET NEUTRAL HEDGE FUNDS
We are using a proprietary data set of equity market neutral hedge funds that are classified as either discretionary or systematic based on the funds’ descriptions. Both types of hedge fund managers aim to have a zero correlation to the stock market, but derive trading decisions differently. Discretionary funds are typically fundamentally focused and employ financial analysts, often former investment bankers or sell-side research analysts. Systematic funds follow models run by quants that tend to have PhDs in computer science or engineering.
Intuitively, we might expect systematic funds to outperform discretionary ones by a consistently increasing margin given the rapid improvements in technology in recent years. However, we observe a similar performance in the period between 2009 and 2020.
In fact, systematic funds have underperformed discretionary funds since 2018 and done especially poorly during the COVID-19 crisis.
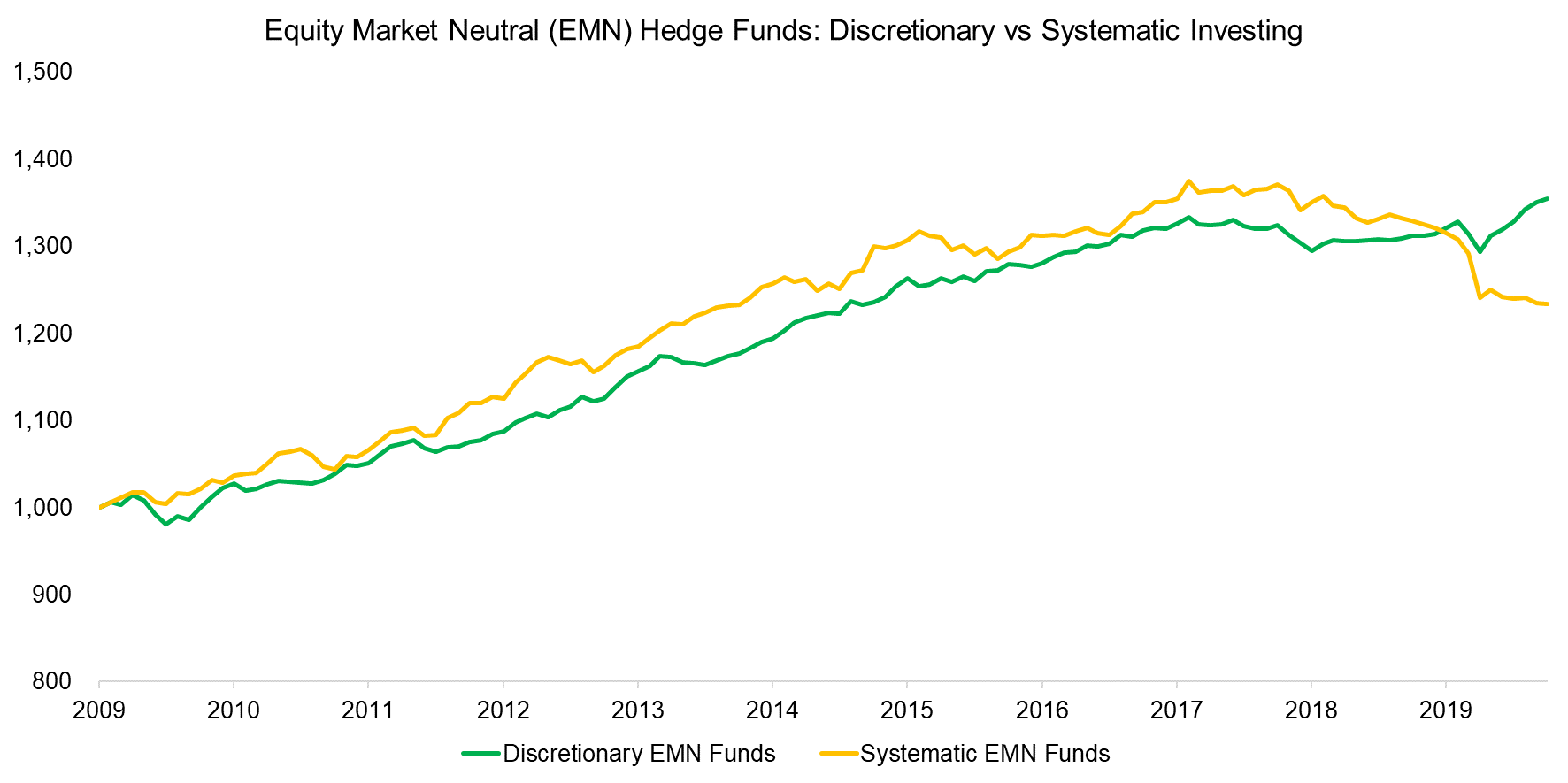
Source: ACE Capital Investment, FactorResearch
One explanation might be that discretionary hedge fund managers adapted quicker to the pandemic, which is a new market event, while systematic models where stuck in the past by following their models which did not have a pandemic within their modelling. However, there is little evidence that humans perform well when markets crash and volatility is high. For example, most US mutual funds underperformed the S&P 500 during the global financial crisis in 2009.
A more data-driven approach to understand the nuances between both types of hedge fund managers is to run a factor exposure analysis to common equity factors. This reveals that systematic funds had higher exposure to Value, Momentum, and Low Volatility factors as well as more negative exposure to the Quality factor. The latter exposure is somewhat unusual, but the positive exposure to factors is expected given that many systematic funds will pursue factor investing.
Value has performed poorly over the last decade and especially poorly over the last two years as the short portfolio included some of the most expensive, but also best-performing stocks like the FAANGs or Tesla. The divergence in the performance of discretionary and systematic funds in recent years can therefore be attributed to different factor exposure.
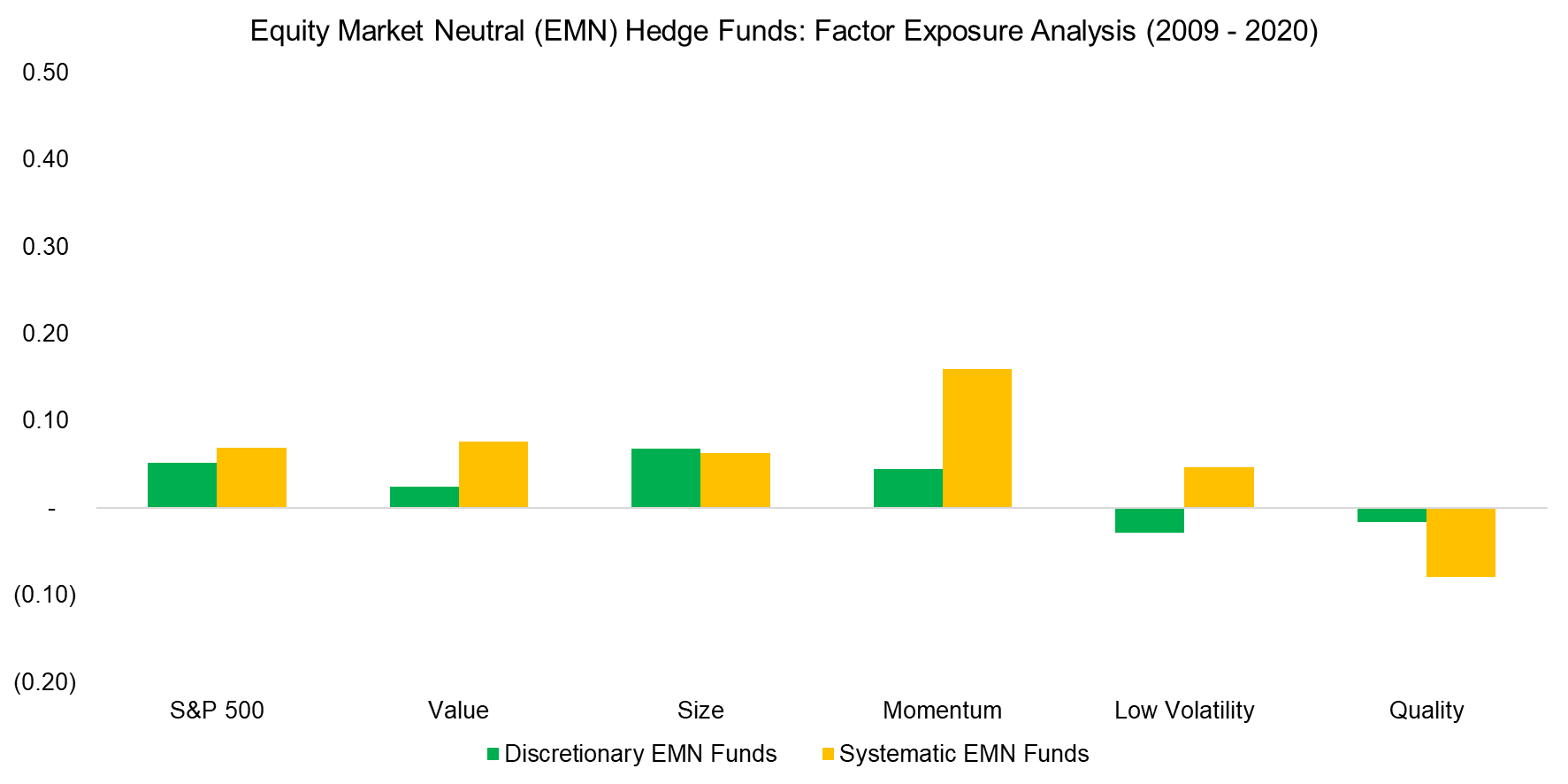
Source: ACE Capital Investment, FactorResearch
HIGHLIGHTING SURVIVORSHIP BIAS
It is worth noting that the data set of equity market neutral hedge funds was first compiled at the beginning of 2020 and then updated in the fourth quarter of the same year. The data set contains only 28 discretionary and 22 systematic funds. More importantly, the data set was created based on the hedge funds being alive in 2020 and does not include ones that performed poorly and were liquidated since 2009, which introduces survivorship bias in the index performance.
We can visualize the significance of survivorship bias by showing two different equity market neutral hedge fund indices, of which one (Eurekahedge) features high and the other (HFRX) low on survivorship bias. We observe a large difference in performance that equates to 4.2% annually.
If our data set would have included all liquidated funds since 2009, then the performance would likely have been much closer to the HFRX than to the Eurekahedge index, which effectively means zero to negative alpha for hedge fund investors over the last decade; this is in line with our findings within our previous research note (read Equity Market Neutral Funds: Powered by Beta?). We can see that many funds that performed poorly during the COVID-19 crisis were liquidated shortly thereafter. Investors have no patience for poorly performing hedge funds.
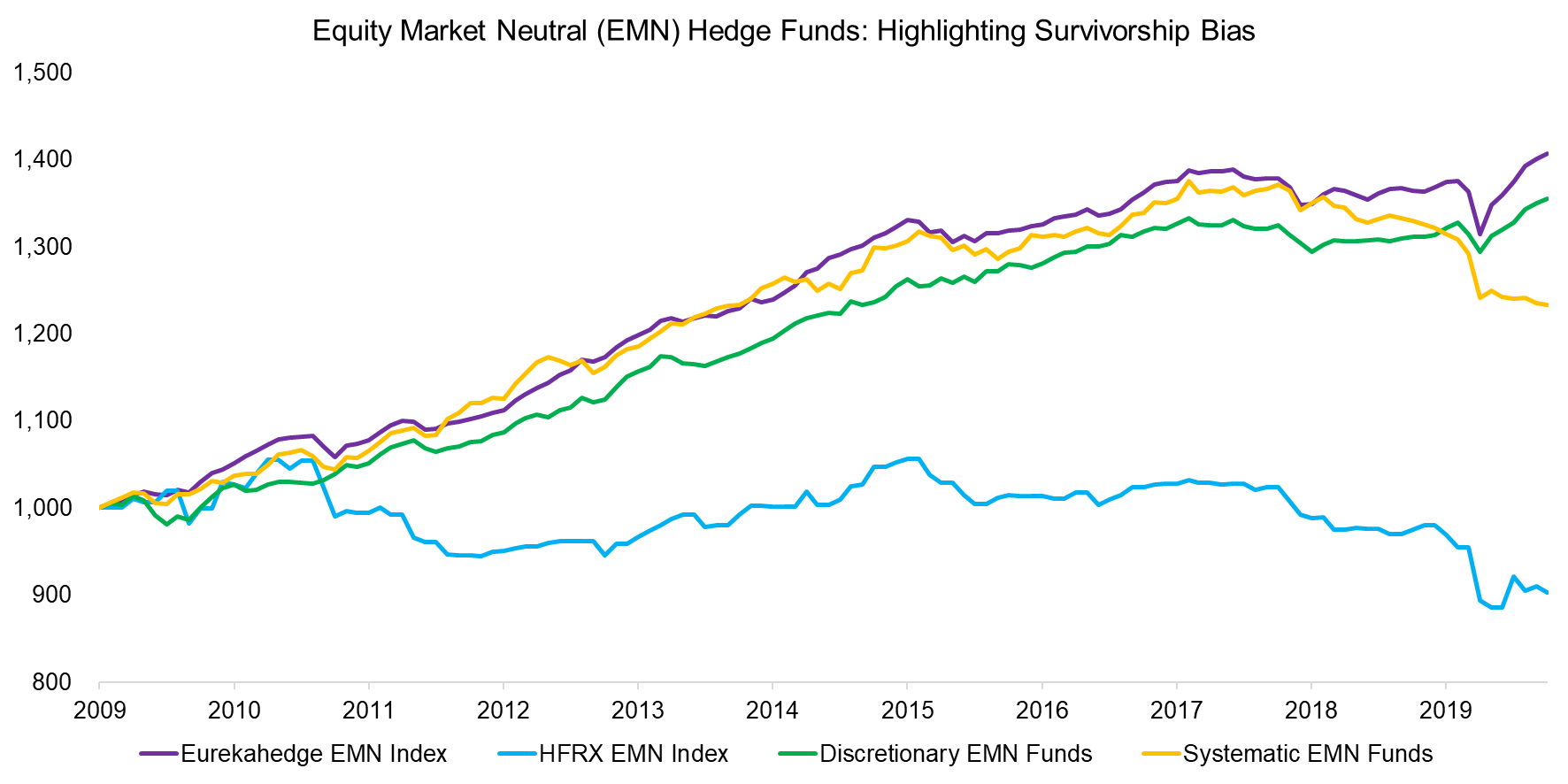
Source: Eurekahedge, HFRX, ACE Capital Investment, FactorResearch
DIVERSIFICATION BENEFITS
Although the performance of discretionary or systematic funds has been poor when adjusted for survivorship bias, they can still be value-adding in asset allocation if they offer low correlations to traditional assets. However, calculating the three-year correlation to the S&P 500 highlights that these funds were highly correlated to the stock market during certain periods over the last decade.
Naturally, this should not have been the case as the beta of an equity market neutral hedge fund should be close to zero at all times. Hedge fund managers, like anyone else, do not know when stock markets crash and running high betas creates the risk of failing to provide capital protection when most needed by capital allocators.
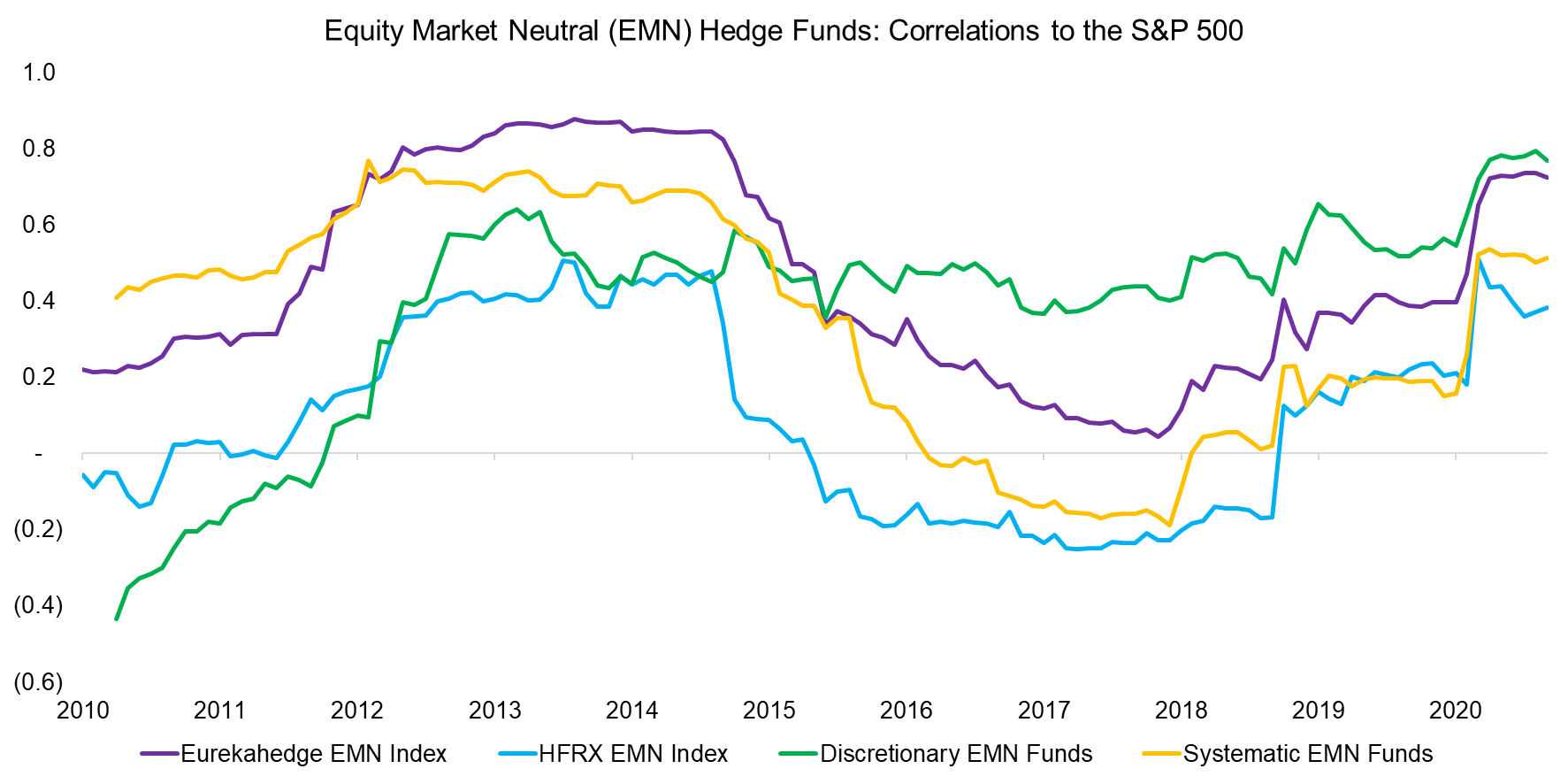
Source: Eurekahedge, HFRX, ACE Capital Investment, FactorResearch
PERFORMANCE CONSISTENCY
However, the analysis can be challenged given that we focused on discretionary and systematic traders on index level. Alpha might be low or zero on average, but some single funds should be able to capture alpha consistently and therefore offer compelling value to capital allocators. The empirical evidence for performance consistency in mutual funds and other asset classes like private equity is low, but perhaps this is different in the hedge fund universe.
We divide the observation period into an in-sample period between 2011 and 2015 and an out-of-sample period between 2016 and 2020. Although the in-sample period does not capture an entire market cycle and largely represents a bull market in equities, it includes various minor stock market corrections, which offered hedge funds the opportunity to demonstrate their capital protection characteristics and generate high risk-adjusted returns.
We calculate the Sharpe ratios of the discretionary funds during the in-sample period and group these into quartiles. The analysis highlights that the top quartile fund produced an average Sharpe ratio of 1.6 in the in-sample period, which is quite impressive. However, in the out-of-sample period, these exceptional funds generated the same Sharpe ratio as the previously worst-performing ones, which highlights a lack of performance consistency.
It is worth highlighting that the Sharpe ratios of all funds were significantly higher in the in-sample than in the out-of-sample period, which is likely explained by the high correlation to the booming stock market in the period between 2012 and 2015. They were essentially powered by beta.
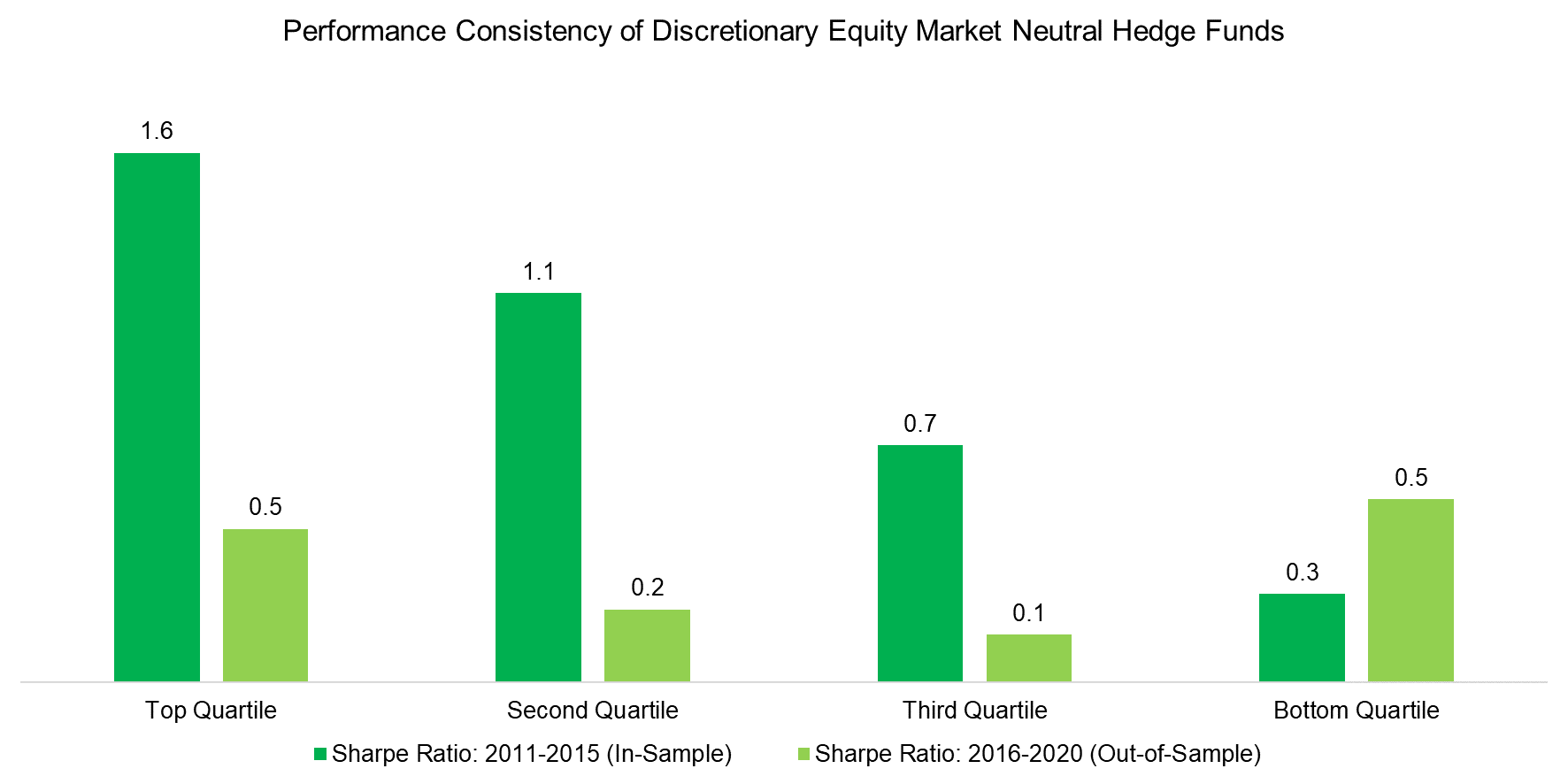
Source: Eurekahedge, HFRX, ACE Capital Investment, FactorResearch
Systematic investing is all about process. If a source of alpha is identified, then a process should be created to repeatedly extract that alpha. Given this process-oriented approach to investing, systematic funds should have a higher performance consistency than discretionary ones. However, this was not the case in our analysis and systematic funds featured an even lower performance consistency. There was no relationship between the Sharpe ratios in the in-sample and the out-of-sample periods.
Although this evaluation of performance consistency is based on a small sample size of funds and covers only a decade of returns, it does highlight the challenge of hedge fund selection.
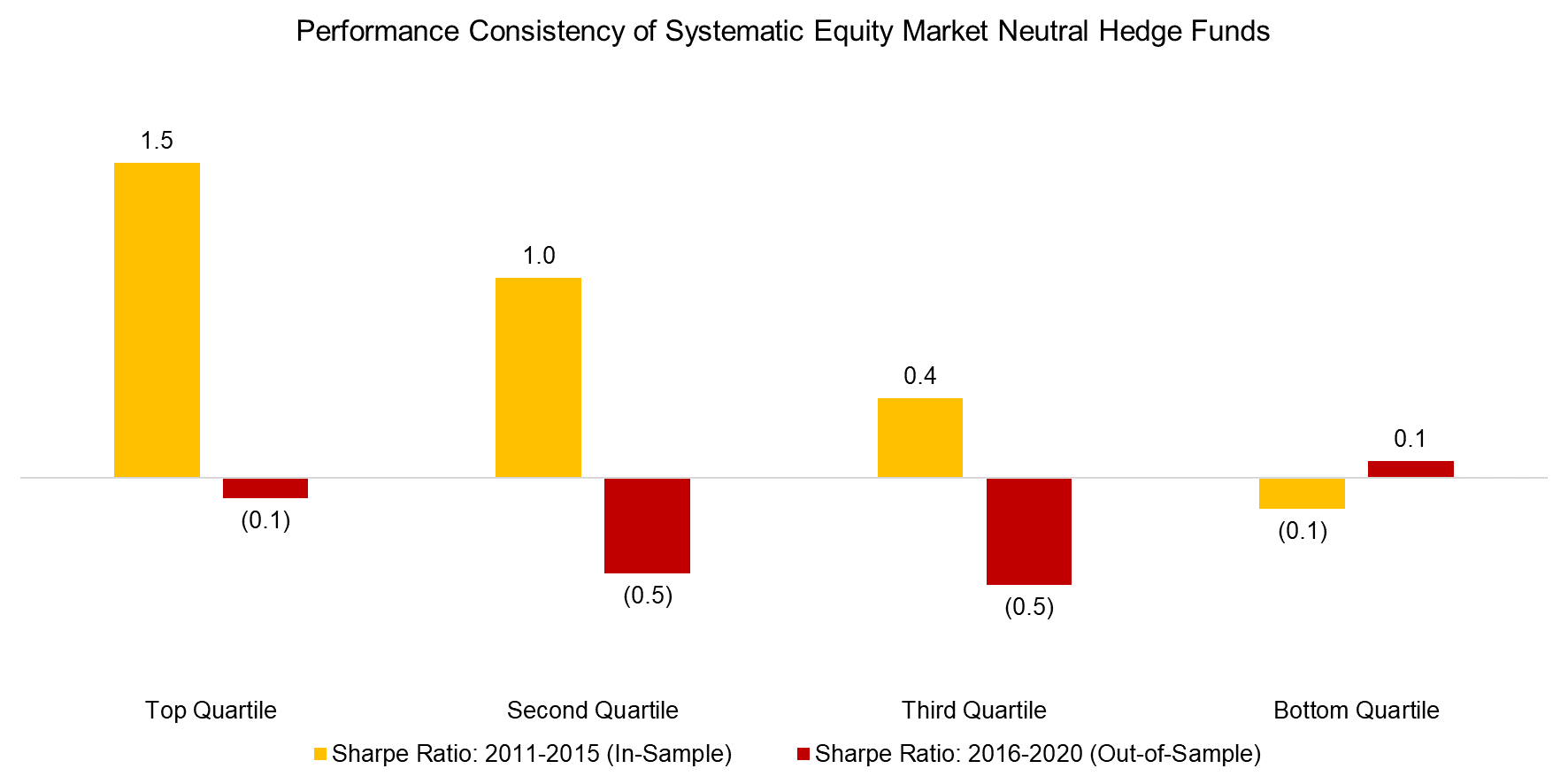
Source: FactorResearch
FURTHER THOUGHTS
Our original quest was to explore if systematic funds generated superior returns to discretionary ones, but despite all the new data, machine learning, and artificial intelligence, they do not seem to have a competitive advantage. There is unlikely a simple answer for explaining this, but multiple reasons.
One explanation is that there are not unlimited sources of alpha. In fact, academic research highlights that most excess returns are explained by a handful of factors. Although discretionary funds approach investing differently, they still mostly exploit the same sources of excess returns as highlighted by the factor exposure analysis.
Secondly, systematic investing has many pitfalls when it comes to creating strategies. Backtesting is often incorrect for various reasons like higher than assumed transaction costs and causes realized returns to be significantly lower than theoretical returns. Most systematic approaches are based on historical data and do not operate well when there are structural changes in markets. Although machine learning and artificial intelligence can theoretically be used to better adapt systematic strategies to new regimes, there is little evidence that they have accomplished this yet.
Finally, although we portrayed both approaches as clearly differentiated, in practice discretionary hedge fund managers use quant screens and systematic ones still need to make plenty of discretionary decisions on strategy design, selection, and implementation.
The best approach is likely to take the best of both worlds by marrying man and machine.
RELATED RESEARCH
Equity Market Neutral Hedge Funds: Powered by Beta?
ABOUT THE AUTHORS
Karl Rogers is the founder of ACE Capital Investments, an Irish-based alternative investments consultant, which specializes in niche, capacity-constrained hedge funds that diversify family office portfolios from their traditional asset class investments. Karl is also the Head of Trading, US Power for RISQ, where he is responsible for building out the proprietary US power trading desk and sits on the firm’s risk committee. Karl also sits in the adjunct faculty for Trinity College Dublin’s M.Sc. in finance program where he is both a guest lecturer in their alternative investment module and supervises the student’s theses. Karl is a market contributor across various industry platforms including CAIA, AlphaWeek, Family Capital Publishing, and the FM Report. He an alternative investment conference speaker and hedge fund panel moderator. Karl has macroeconomic research published by a top-tier academic journal and is a former hedge fund manager and commodity trader across Ireland and North America.
Connect with me on LinkedIn.
Nicolas Rabener is the Founder of Finominal, which empowers investors with data and technology to analyze and improve their portfolios. Previously he created Jackdaw Capital, an award-winning quantitative hedge fund. Before that Nicolas worked at GIC and Citigroup in London and New York. Nicolas holds a Master of Finance from HHL Leipzig Graduate School of Management, is a CAIA charter holder, and enjoys endurance sports (100km Ultramarathon).